Backed by Y Combinator
RAG that intelligently adapts to your use case, data, and queries
Supercharge your AI applications with Agentic RAG
From the institutions you trust
Created by scientists from world-class institutions
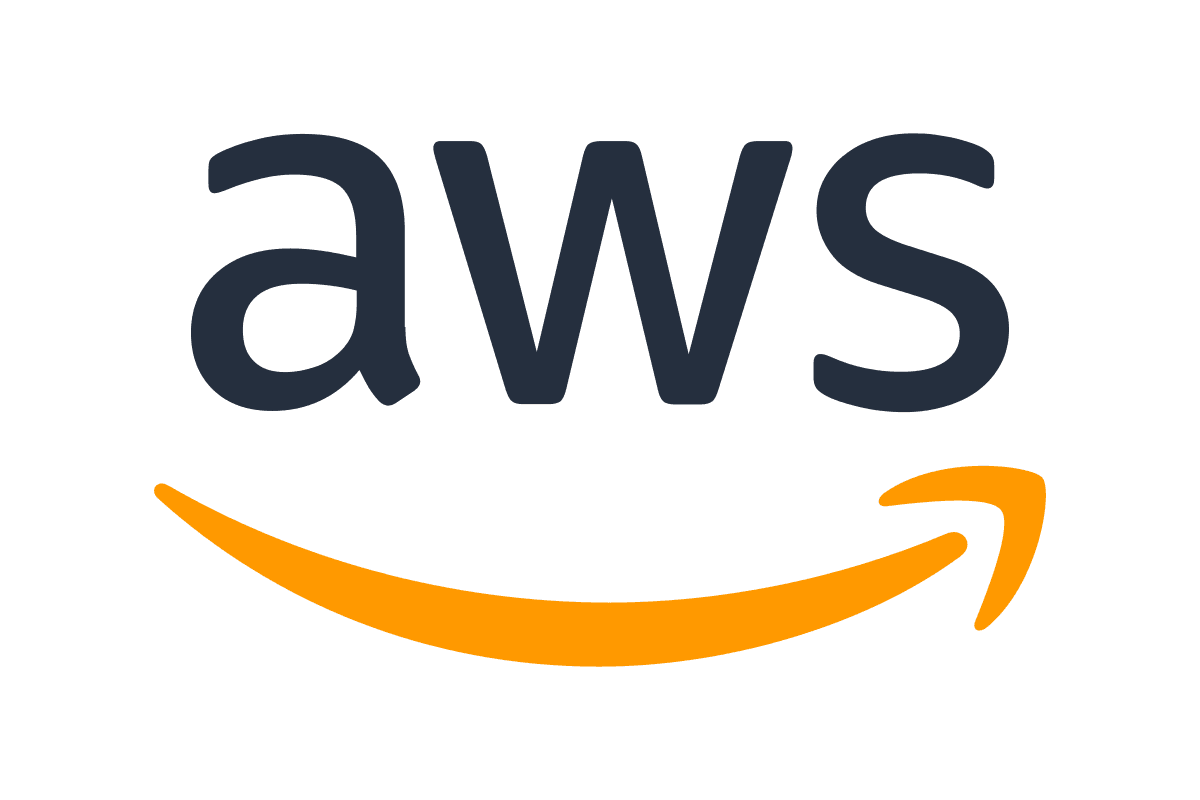
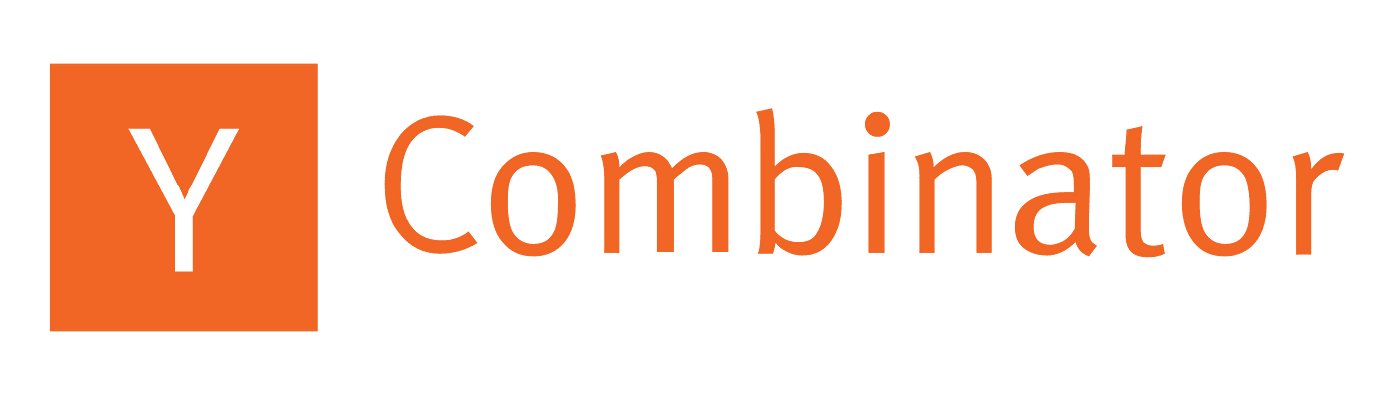
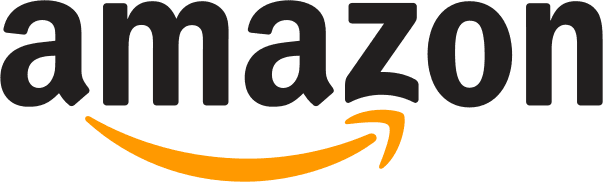
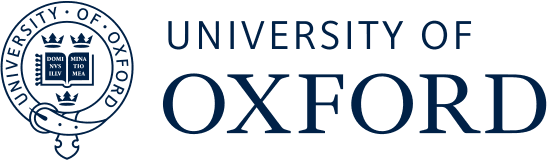
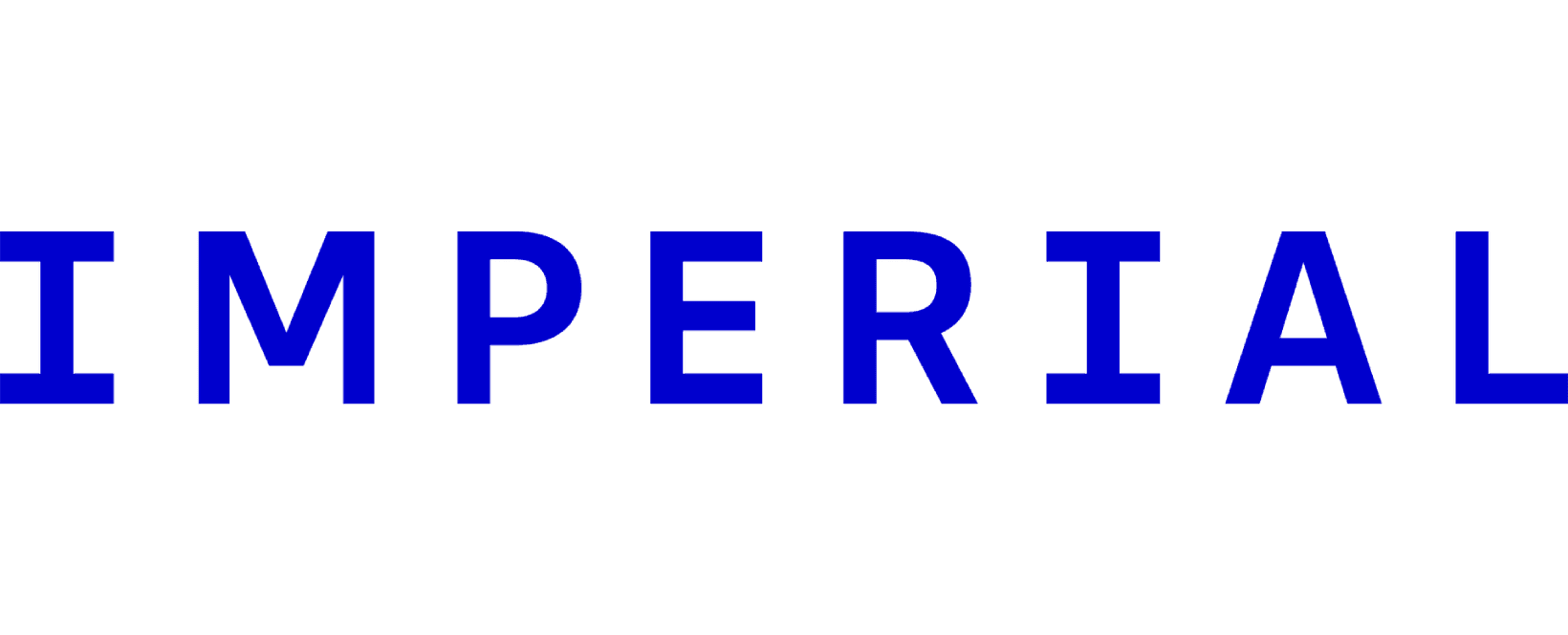
What makes us unique
Promptable GraphRAG
Circlemind empowers you to create sophisticated RAG pipelines in plain English
Our technology
Vector Databases + Knowledge Graphs
You'll never need to build RAG from scratch again — RAG that works amazingly out-of-the-box
Always Self-Improving
Naive RAG uses static representations and does not evolve over time. Our GraphRAG learns from every information and interaction. It constantly re-arranges its memories to serve your specific use case as best as possible.
Multi-Hop Retrieval
Naive RAG fails to combine stored information effectively. Our GraphRAG can reason over memories and retrieve the most relevant information seamlessly.
Whole Dataset Reasoning
Naive RAG struggles with queries such as “top 5 issues customers face.” Our GraphRAG understands your data in aggregate and answers these questions effectively.
Dynamic Data
Naive RAG relies on static embeddings, which limit its ability to model information that evolve over time. In contrast, our GraphRAG can store evolving information, allowing for dynamic adaptation and improved context.
Needle in a Haystack
Naive RAG struggles to capture the nuances of meaning. Our GraphRAG navigates its knowledge graph and finds the most appropriate information just like your brain does.
Codebases
Naive RAG treats data as disjointed pieces, making it difficult to comprehend the overall structure. Our GraphRAG understands the interconnections between components.
Frequently Asked Questions
Can't find what you're looking for? Don't hesitate to contact us for more information
What is Agentic RAG?
Agentic RAG is a framework that incorporates the concept of agents to enhance the capabilities and functionality of the retrieval pipeline. Circlemind uses agentic GraphRAG to analyze, understand, and retrieve your data as it best suits your specific use case.
What do you mean by promptable?
You can control Circlemind's graph construction by describing in English the type of data in use, the domain, the desired behaviour, examples of queries, and so on. Imagine Circlemind like an engineer on your team that will design and implement the best possible knowledge graph based on your descriptions and data.
Our AI will turn your words in a fully functional RAG system that works right out-of-the-box.
When should I use GraphRAG?
GraphRAG is particularly advantageous in scenarios involving domain-specific, dynamic information, such as evolving knowledge, and nuanced context retrieval. Our system outperforms naive RAG by up to 80% in accuracy in applications requiring deep data analysis, domain understanding, and integration of multiple data points. On the flip side, naive RAG is ideal for low-latency use cases involving mostly static data, and with no requirements for high-precision context retrieval.
How can I contact you?
You can reach us at [email protected]
Pricing
Community Edition
Free
Small or hobby projects
Self-hosted
Open-source
Business
$300
per month
Fully managed
Graph visualizer and debugger
10,000 requests included
PDFs ingestion
Autoscaling
Unlimited graphs
Dedicated Slack channel
Enterprise
Custom
Data integrations (e.g. S3, Slack, Salesforce, etc)
White Glove Onboarding Call
VPC and On Prem Deployments
BAA Agreements
Zero Data Retention Agreements
SSO and SAML Authentication
Don't waste time on RAG
Let our AI create the best solution for your use case, data, and questions